Graph convolutional network-based semi-supervisedfeature classification of volumes
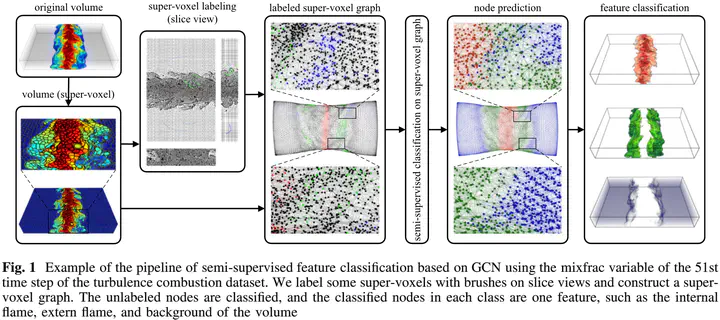
Feature classification has always been one of the research hotspots in scientific visualization.However, conventional interactive feature classification methods rely on prior knowledge and typicallyrequire trial and error, whereas feature classification based on data mining is generally based on localfeatures; therefore, obtaining good results with traditional methods is difficult. In this paper, we first map avolume to the super-voxel graph using a 3D extension of the simple linear iterative clustering algorithm andthen construct a graph convolutional neural network to implement node classification in a semi-supervisedway, i.e., a small number of user-labeled super-voxels. We transform the feature classification of a volumeinto the classification task of nodes of a super-voxel graph, which is a novel approach and broadens theapplication scope of graph neural netwo rk to volumes. Experiments on different volumes have demonstratedthe strong learning ability and reasoning ability of the proposed method.