Exploring Evolution of Dynamic Networks via Diachronic Node Embeddings
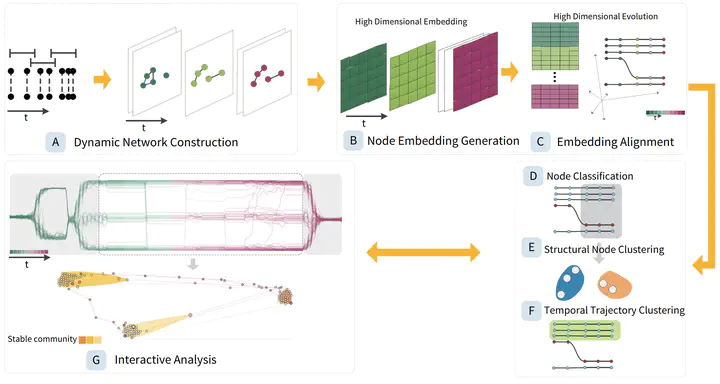
Dynamic networks evolve with their structures changing over time. It is still a challenging problem to efficiently explore the evolution of dynamic networks in terms of both their structural and temporal properties. In this paper, we propose a visual analytics methodology to interactively explore the temporal evolution of dynamic networks in the context of their structure. A novel diachronic node embedding method is first proposed to learn latent representations of the structural and temporal features of nodes in a vector space. Diachronic node embeddings are then used to discover communities with similar structural proximity and temporal evolution patterns. A visual analytics system is designed to enable users to visually explore the evolutions of nodes, communities, and the network as a whole in terms of their structural and temporal properties. We evaluate the effectiveness of our method using artificial and real-world dynamic networks and comparisons with previous methods.